Amy Sprague
March 29, 2024
ISE associate professor Chaoyue Zhao, a recipient of the prestigious NSF CAREER Award, is revolutionizing the field of energy infrastructure control. Her innovative work combines reinforcement learning (RL), mathematical optimization, and expert feedback to enhance the resilience and efficiency of automated control systems within the energy sector.
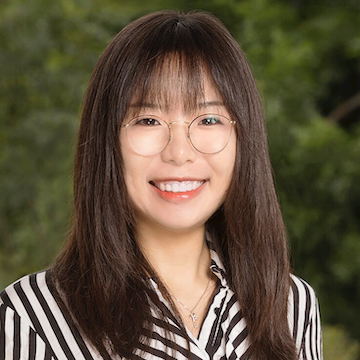
Associate Professor Chaoyue Zhao
What is reinforcement learning?
Reinforcement learning (RL) is a machine learning technique that trains the artificial intelligence (AI) agent to learn optimal behavior through trial and error in an interactive environment. Unlike other machine learning approaches, RL does not rely on labeled input/output pairs but instead uses rewards and punishments as signals for positive and negative behavior. The agent explores the environment, takes actions, and learns from the feedback it receives.
Integrating reinforcement learning with expert knowledge
Zhao's research addresses key challenges in applying RL to complex energy infrastructure problems. These challenges include safety, sample efficiency, and optimality. Her project, which is receiving over $500,000 from NSF, seeks to overcome these challenges through two main approaches: excavating unexploited information and integrating expert knowledge.
To improve safety, sample efficiency, and optimality, she is developing a modeling methodology that preserves valuable information from training data. By applying optimistic distributionally robust optimization (ODRO) principles, she aims to remove distributional assumptions and improve solution optimality. This approach also enhances sample efficiency by reducing the rejection rate during policy updates.
The project integrates expert knowledge with RL agents. By constructing an expert-guided framework, human expertise prevents unsafe operations and guides RL agents' learning. This framework incorporates expert demonstrations and in-the-loop feedback, expediting the learning and exploration process while boosting sample efficiency. Also, an automatic detection mechanism for action safety is proposed, alerting human overseers to intervene when necessary.
Applications
The expert-guided policy optimization framework developed through this research has wide-ranging applications. First and foremost is the control of heating, ventilation, and air conditioning (HVAC) systems of residential and commercial buildings. Zhao notes that “This is a critical part of the energy infrastructure and is undergoing perhaps the most radical changes to automatic control. The goal is an energy-efficient HVAC control strategy that can effectively reduce energy consumption while still maintaining comfortable indoor conditions.”
Another important application is demand response (DR), a method for reducing peak load and mitigating uncertainties in the electricity market. Zhao's research offers a promising solution for distribution-level DR, addressing the challenges posed by a large number of participants and the resulting policy instability. The research aims to identify optimal dynamic pricing policies to enhance the effectiveness of DR.
Another important application is AC optimal power flow (OPF), which is crucial for power system operation. Zhao's research focuses on solving the highly non-convex and non-linear AC-OPF problem, considering the challenges posed by renewable energy integration. The on-policy RL method developed in this research offers superior sample efficiency and improved reliability, ensuring the feasibility and stability of control processes.
ISE interim chair Cynthia Chen sees this project as a great step toward energy sustainability, “Professor Zhao’s research is a significant step forward in enhancing the control systems of energy infrastructure. By integrating reinforcement learning, mathematical optimization, and expert guidance, her work promises to revolutionize the energy sector, ensuring a more resilient and efficient future.”